Over the past decade, artificial intelligence has dramatically evolved, making significant strides in natural language processing (NLP), deep learning, speech recognition, and pattern recognition. These advancements have led to the creation of intelligent systems capable of understanding, processing, and generating human-like text—one of which is the Sequence-to-Sequence (Seq2Seq) model.
This architecture is a foundational component behind Google Translate, voice assistants like Google Assistant, and many modern chatbots.
In this article, we’ll dive deep into the Seq2Seq model—what it is, how it works, its architecture, applications, types, and the challenges it faces in deployment. Whether you’re a data science enthusiast or a machine learning practitioner, this guide will provide a comprehensive overview of the model that transformed machine translation and conversational AI.
📌 Learning Objectives
By the end of this article, you’ll understand:
- What a Seq2Seq model is and how it works.
- Real-world applications of Seq2Seq in AI systems.
- The architecture and types of Seq2Seq models.
- Challenges faced when training and deploying these models.
- The evolution of machine translation from rule-based to neural networks.
🧠 What is a Seq2Seq Model?
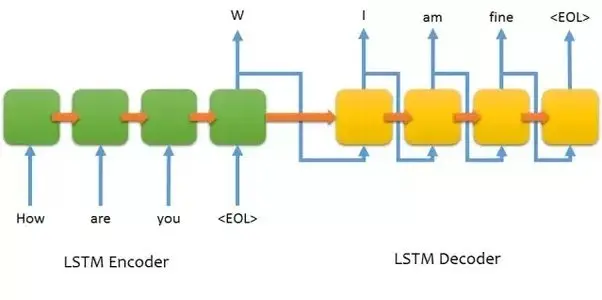
At its core, a Seq2Seq model (Sequence-to-Sequence model) is a deep learning architecture used in tools like Google Tradutor to translate sequences from one language to another. With its encoder-decoder structure, it enables language models to produce grammatically coherent and context-aware outputs.
At its core, a Seq2Seq model (Sequence-to-Sequence model) is a type of neural network architecture that is designed to convert sequences from one domain (e.g., English text) to sequences in another domain (e.g., French text). These sequences could vary in length and structure, which adds complexity to the task.
Traditionally, machine translation involved rule-based or phrase-based approaches. These systems often failed to preserve grammar and contextual meaning. With Seq2Seq models, deep learning introduced a more flexible and powerful method that can learn patterns, syntax, and semantics directly from large corpora of data.
The concept of Seq2Seq was introduced by researchers at Google in 2014. They leveraged Recurrent Neural Networks (RNNs), especially Long Short-Term Memory (LSTM) networks, to encode input sequences and decode them into target sequences. This architecture brought a revolution in machine translation, making translations more grammatically accurate and context-aware.
🌍 Real-World Applications of Seq2Seq Models
Seq2Seq models are used in various AI applications where the output is a sequence dependent on the input. Let’s explore the most prominent use cases:
1. Machine Translation (e.g., Google Translate and Google Tradutor)
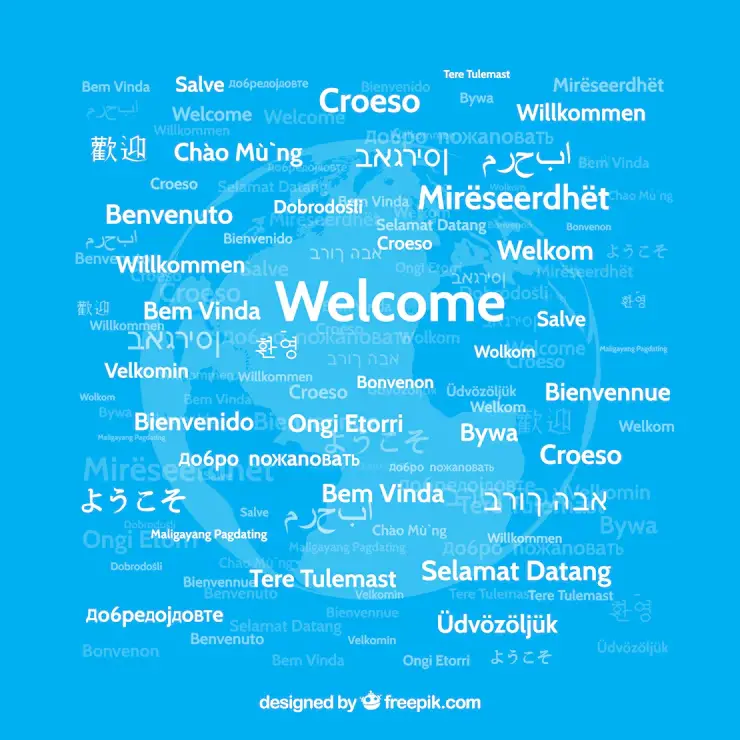
The most prominent application of Seq2Seq is in machine translation tools like Google Translate and Google Tradutor. These services rely on advanced neural networks to convert entire phrases and paragraphs from one language to another, rather than translating word-for-word. This results in smoother, more natural-sounding translations.
2. Chatbots and Conversational AI
AI chatbots use Seq2Seq models to understand user queries and generate relevant responses. In platforms like Google Assistant, Amazon Alexa, or customer support bots, the model takes the user’s message as input and produces a natural language reply.
3. Speech Recognition
Speech-to-text systems, like those used in voice typing or digital dictation, convert spoken words into written text. Here, the input is a time series of audio frames, and the output is a sequence of characters or words.
Companies like Nuance, Apple (Siri), and Uniphore use speech recognition engines built on top of Seq2Seq frameworks.
4. Video Captioning
Automatic caption generation for videos requires understanding both audio and visual content to generate descriptive text. Platforms like YouTube and Netflix use these models to improve accessibility.
5. Text Summarization
Seq2Seq models can read a long piece of text and summarize it into a shorter version while preserving meaning. This has applications in news aggregation, content curation, and legal document analysis.
6. Question Answering Systems
In education and customer service, models take in a query and generate a detailed answer. These systems are also built on Seq2Seq architectures with added components like attention mechanisms.
🧩 How Does the Seq2Seq Model Work?
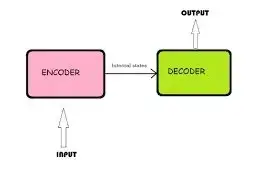
The magic behind Seq2Seq lies in its Encoder-Decoder architecture, often augmented with RNN variants like LSTM or GRU (Gated Recurrent Unit). Let’s break this down:
🔹 Encoder
- The encoder reads the input sequence (e.g., a sentence in English) one word at a time.
- Each word is converted into an embedding and passed through RNN/LSTM layers.
- The final hidden state of the encoder serves as a compressed context vector—a numerical summary of the input.
🔹 Decoder
- The decoder takes the context vector as its starting point.
- It generates the output sequence (e.g., a sentence in French) word by word.
- Each output word is predicted based on the current hidden state, previous output, and the context vector.
The decoder continues this process until it generates an end-of-sequence token (<EOS>
), signaling the completion of the output.
🔄 RNN, LSTM, and GRU in Seq2Seq
Seq2Seq models were originally built using Recurrent Neural Networks (RNNs), which are ideal for processing sequential data. However, RNNs suffer from vanishing gradients, making them ineffective for long sequences.
To solve this, two major improvements were introduced:
- LSTM (Long Short-Term Memory): Introduced memory cells and gating mechanisms to retain long-term dependencies.
- GRU (Gated Recurrent Unit): A simplified version of LSTM with fewer gates but comparable performance.
Most modern Seq2Seq models use either LSTM or GRU, depending on the specific use case.
🔍 Types of Seq2Seq Models
Seq2Seq models have evolved over time. Below are the main types:
1. Vanilla Seq2Seq Model
- This is the original version using LSTM or GRU for both the encoder and decoder.
- It uses a single context vector passed from encoder to decoder.
- Limitation: It struggles with long sequences as the single context vector may not hold all relevant information.
2. Attention-Based Seq2Seq Model
- Introduced to overcome the limitation of fixed context vectors.
- Instead of relying on the last hidden state alone, the attention mechanism allows the decoder to access all hidden states of the encoder.
- The decoder “attends” to different parts of the input sequence at each decoding step, making translation or output generation more accurate.
Popular variants:
- Bahdanau Attention (Additive)
- Luong Attention (Multiplicative)
3. Transformer Models
Though technically a departure from classic RNN-based Seq2Seq models, transformers are inspired by Seq2Seq principles. They use self-attention mechanisms and allow parallel computation, making them faster and more efficient.
Transformers form the basis of BERT, GPT, and T5, which power modern NLP tasks today.
🔧 Challenges Faced by Seq2Seq Models
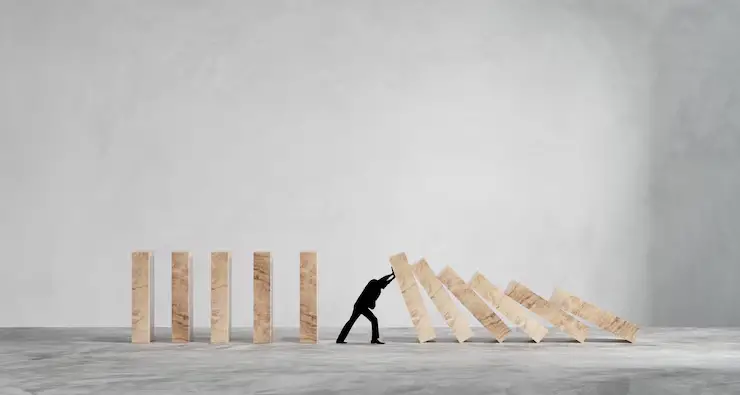
Despite their capabilities, Seq2Seq models have several challenges:
1. Computational Cost
Training large Seq2Seq models, especially with attention mechanisms or transformers, requires significant computational resources and memory.
2. Overfitting
If not regularized properly, models may memorize training data and fail to generalize to unseen examples.
3. Handling Long Sequences
In vanilla models, the context vector can’t retain full meaning for long inputs, leading to degraded output quality.
4. Unknown Words or Tokens
Out-of-vocabulary (OOV) words can be hard to handle. Modern models use subword tokenization (e.g., Byte Pair Encoding) to mitigate this.
5. Lack of Interpretability
It’s often difficult to understand how and why a Seq2Seq model makes certain decisions, especially in the absence of attention mechanisms.
🛠 Enhancements and Best Practices
To improve the performance and reliability of Seq2Seq models, practitioners use several strategies:
- Beam Search for better output sequence generation.
- Dropout and Layer Normalization for regularization.
- Subword Tokenization to handle unknown words.
- Transfer Learning with pre-trained language models.
- Hybrid Models that combine CNNs for feature extraction and RNNs for sequence modeling.
💡 Conclusion
The Seq2Seq model has been a cornerstone in the development of modern AI systems for natural language processing. From powering Google Translate to enabling voice assistants, chatbots, and text summarizers, this model has demonstrated remarkable versatility and performance in sequence-based tasks.
As technology advances, attention mechanisms and transformers have begun to outshine the original Seq2Seq architecture. However, the fundamental encoder-decoder concept still plays a vital role in NLP.
Whether you’re building a chatbot, creating automated subtitles, or designing a machine translation engine, understanding Seq2Seq architecture provides a strong foundation for innovation.